BJUI journal prizes
Every year the BJUI awards three prizes to trainee urologists who have played a significant role in contributing to the work published in the journal. The prizes go towards travel costs enabling the trainees to visit international conferences. In 2020, due to the coronavirus pandemic leading to the cancellation of many of these conferences, the usual prize-giving ceremonies have not taken place so here we are introducing you to the prize winners and their work. We hope they will be able to spend their prize money in 2021.
Global prize
This is awarded to authors who are trainees based anywhere in the world other than the Americas and Europe. Usually presented at the USANZ annual meeting. In 2020 the prize was awarded to Sho Uehara for his work on artificial intelligence in prostate cancer diagnosis.
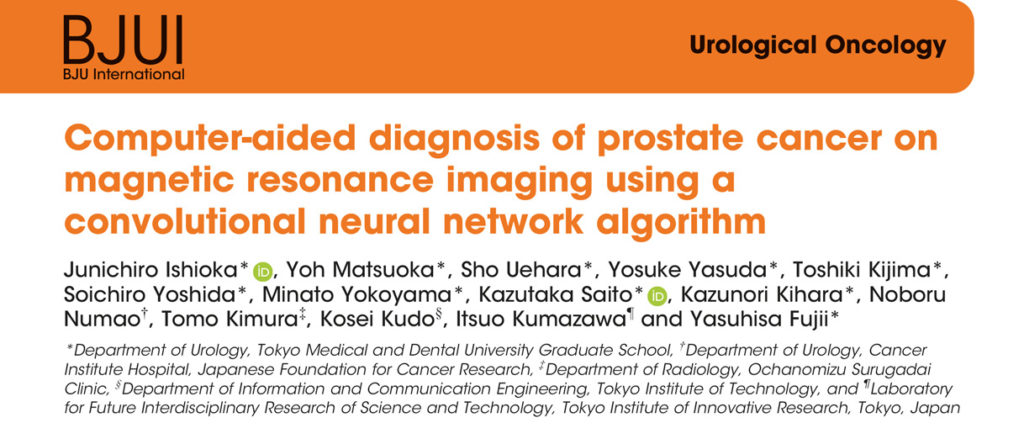
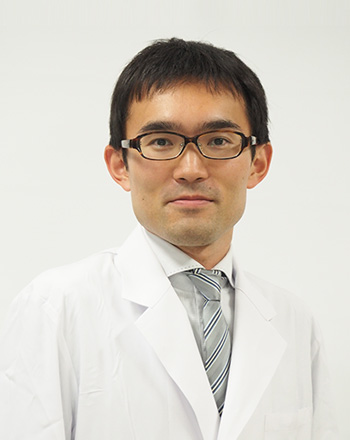
Assistant professor, Department of Urology
Tokyo Medical and Dental University
Email: [email protected]
Sho Uehara received a Ph.D. from the graduate school of Tokyo Medical and Dental University, Tokyo, Japan, in 2018. He is now working as a urologist and an assistant professor at the university hospital. His research interests include prostate cancer diagnostics, and utilization of machine learning for them.
Membership of academic societies:
JUA (The Japanese Urological Association), EAU (European Association of Urology) and AUA (American Urological Association)
Coffey-Krane prize
The Coffey-Krane prize is awarded to an author who is a trainee based in The Americas. Normally presented at the AUA annual conference. Dr Nathan Wong received this year’s award for his work on using machine learning to predict biochemical cancer recurrence following prostatectomy.
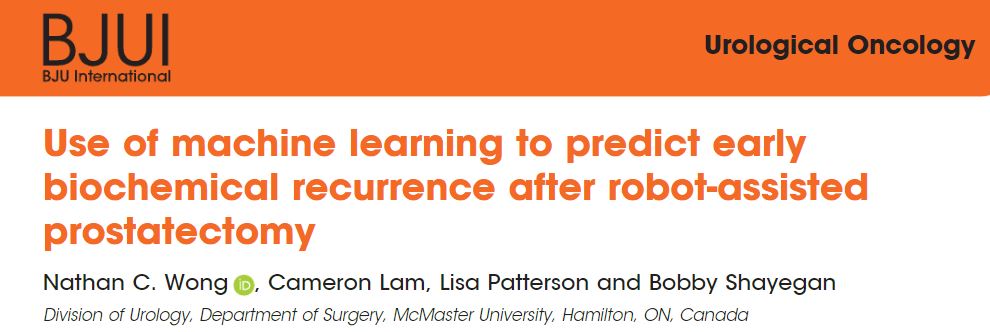
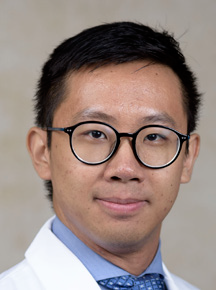
Associate Professor
Westchester Medical Center and New York Medical College
Dr Nathan Wong is an assistant professor and associate program director in the Department of Urology at Westchester Medical Center and New York Medical College. He specializes in urologic oncology and robotics surgery. His main interests are in technology, clinical trials and surgical education. He completed a Society of Urologic Oncology fellowship at Memorial Sloan Kettering Cancer Center in New York City and urology residency at McMaster University in Hamilton, Ontario in Canada.
John Blandy prize
This prize is for authors who are trainees based in Europe. Presented at the BAUS annual conference; the winner gives a presentation. This year the prize went to Nicholas Raison for his work on a RCT on cognitive training in robotic surgery.
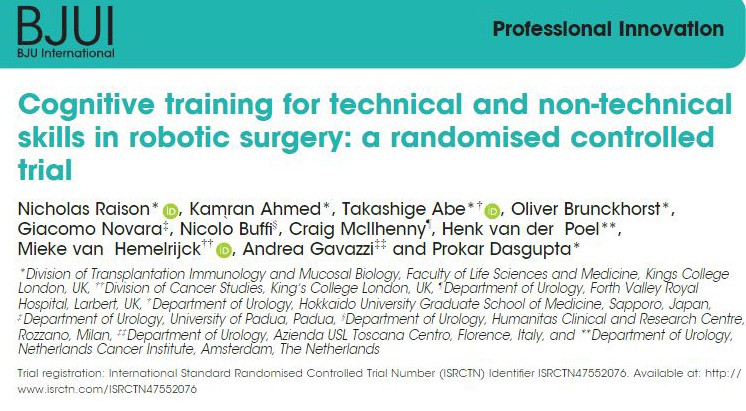
Nicholas Raison is Vattikuti fellow at the MRC Centre for Transplantation and Mucosal Cell Biology, King’s College London and a Urology Specialist Registrar in the London Deanery.